Presentation
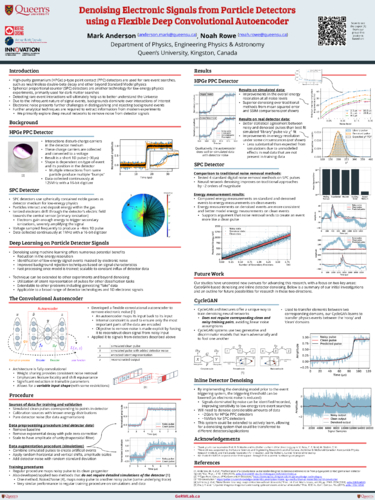
P16 - Denoising Electronic Signals from Particle Detectors Using a Flexible Deep Convolutional Autoencoder
Presenters
DescriptionIn this work, we present the use of a deep convolutional autoencoder to denoise signals from particle detectors. The study of rare particle interactions is crucial in advancing our understanding of the Universe. However, the presence of electronic noise makes signal events difficult to distinguish from backgrounds, especially due to the infrequent nature of the interactions we are searching for. We begin with our recently published results on germanium detectors demonstrating that deep learning is more effective at removing noise than traditional approaches, while still preserving the underlying pulse shape well. We show that our approach also has practical implications on data storage and processing efficiency. To extend on our published work, we explored additional deep learning-based methods for signal denoising and modeling. We then present our simulations on implementing these algorithms at the hardware level directly for real-time denoising and data selection. Finally, we demonstrate that our approach is broadly applicable to other detector technologies and one-dimensional electronic signals.
TimeMonday, June 2611:20 - 11:50 CEST
LocationDavos
Session Chair
Event Type
Poster