Presentation
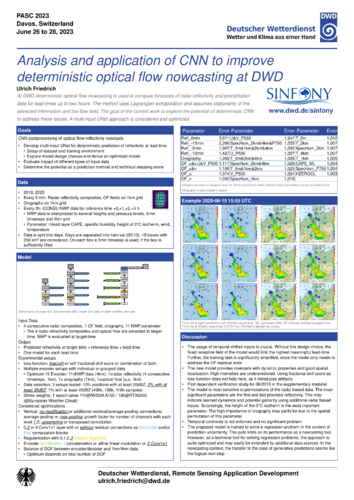
P08 - Analysis and Application of CNN to Improve Deterministic Optical Flow Nowcasting at DWD
Presenter
DescriptionOptical flow based nowcasting is essential for several operational productions at DWD, including time critical warnings. Precipitation and radar reflectivity nowcasts are produced every 5 minutes with a 5 minute stepping up to 2h lead time. The method assumes stationarity of the input data. It is a deterministic advection scheme without dynamic properties and does not take advantage of additional data sources.Recently, machine learning techniques were tested in nowcasting. Deterministic methods struggle to predict high-intensity values and become blurry for larger lead times. In this presentation we explore the potential of deterministic convolutional neural networks (CNN) to improve the operational nowcasting at DWD. A two-year dataset consisting of radar, NWP and orography data is used for training modified UNet based neural networks. The goals are to understand the technically limitations of the approach as well as the impact of the additional input data. Clever data manipulation and adaption of the network architecture to its properties are key. An impact study for the input data is performed. We explore combination methods for several data encoders, additional computation blocks for more nonlinearity and loss functions with spatial context. Baselines for comparison are the operational nowcasting at DWD and CNN approaches from literature.
TimeMonday, June 2611:20 - 11:50 CEST
LocationDavos
Session Chair
Event Type
Poster