Presentation
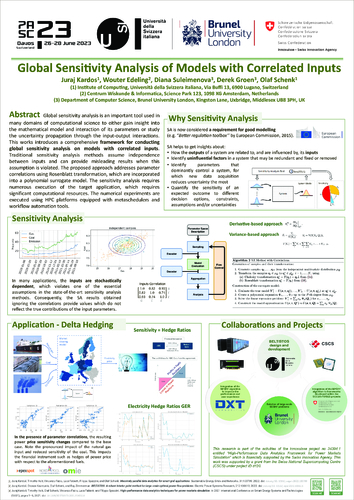
P26 - Global Sensitivity Analysis of High-Dimensional Models with Correlated Inputs
Presenter
DescriptionGlobal sensitivity analysis is an important tool used in many domains of computational science to either gain insight into the mathematical model and interaction of its parameters or study the uncertainty propagation through the input-output interactions. This works introduces a comprehensive framework for conducting global sensitivity analysis on models with correlated inputs. Traditional sensitivity analysis methods assume independence between inputs and can provide misleading results when this assumption is violated. The proposed approach addresses parameter correlations using transformations such as Rosenblatt and Cholesky, which are incorporated into a polynomial surrogate model used to evaluate sensitivity indices. The effectiveness of the method is demonstrated through numerical experiments, which are conducted using the EasyVVUQ framework. The sensitivity analysis requires numerous execution of the target application, which requires significant computational resources. The numerical experiments are thus executed using HPC platforms equipped with a metascheduler and workflow automation tools. The results of these experiments are discussed and provide insights into the impact of correlated inputs on the sensitivity analysis.
TimeMonday, June 2611:20 - 11:50 CEST
LocationDavos
Session Chair
Event Type
Poster