Presentation
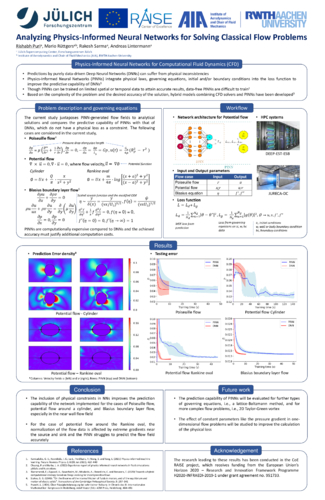
P09 - Analyzing Physics-Informed Neural Networks for Solving Classical Flow Problems
Presenter
DescriptionThe application of Neural Networks (NNs) has been extensively investigated for fluid dynamic problems. A specific form of NNs are Physics-Informed Neural Networks (PINNs), which incorporate physics-based embeddings to account for physical laws. In this work, the performance of PINNs is compared to that of DNNs with respect to accuracy. Therefore, results obtained from PINNs and DNNs are compared to analytical solutions of four classical flow problems - Poiseuille flow, potential flow around cylinder and Rankine oval, and Blasius boundary layer flow. The findings show that the PINNs provide more accurate representations of the flow fields than their DNN counterpart for potential flow around a cylinder and Blasius boundary layer flow. The investigations show that in some flow problems, inclusion of information on problem physics, governing equations, and boundary conditions in the loss function of an NN can improve prediction accuracy of NNs. Since PINNs are computationally expensive compared to DNNs, it is also investigated if the accuracy achieved with PINNs over DNNs is significantly high to justify the additional computation costs that are associated with their training.
TimeMonday, June 2611:20 - 11:50 CEST
LocationDavos
Session Chair
Event Type
Poster